Propelled by the growth in demand for artificial intelligence-enabled applications, the past decade has witnessed the emergence of Collaborative Cyber-Physical Learning Systems (CCPLS). CCPLS carry out distributed, learning-based processing tasks through coordination among Cyber-Physical System (CPS) devices, and are envisioned to provide critical functionality across the commercial and defense sectors in the next several years. However, the data generated by CCPLS is often large-scale, high-dimensional, heterogeneous, and time-varying, which poses critical challenges for intelligence modeling. Concurrently, unmanned vehicles, in particular Unmanned Aerial Vehicles (UAVs), have shown promise of scaling up information-sharing in CCPLS, especially in under-served regions such as rural areas. The project's novelties are in establishing a concrete foundation for UAV-CCPLS integration that unifies the associated learning, networking, and communication design aspects around appropriate intelligence metrics. The project's impacts are the development of UAV-assisted CCPLS for smart agriculture tasks, as well as advancing the manufacturing of UAVs and other unmanned vehicles tailored for CCPLS. Project outcomes will be disseminated by releasing open-source software and research videos and tutorials. The investigators will further engage in Curriculum development, diversity, and outreach activities including mentoring undergraduate researchers. Research investigations center around three interconnected thrusts. Thrust 1 develops a novel UAV-assisted intelligence framework for CCPLS and introduces a precise, task-oriented notion of data dynamics and heterogeneity. Additionally, this thrust develops a `learning for learning? framework that aims to predictively estimate the rate of data dynamics. Thrust 2 investigates methodologies for jointly optimizing resource utilization and intelligence quality through co-design of UAV trajectories and UAV-to-CPS network establishment. The data dynamics framework from Thrust 1 is integrated into this design through an online, network-aware sequential decision-making framework. Finally, Thrust 3 develops CCPLS communication protocols based on learning-aware uplink and downlink wireless beamformers and over-the-air aggregation methods. These protocols are tailored to the specific needs of the UAV-assisted learning systems, e.g., the transmission of noisy information over UAV-to-UAV and UAV-to-access point communication links. This award reflects NSF's statutory mission and has been deemed worthy of support through evaluation using the Foundation's intellectual merit and broader impacts review criteria.
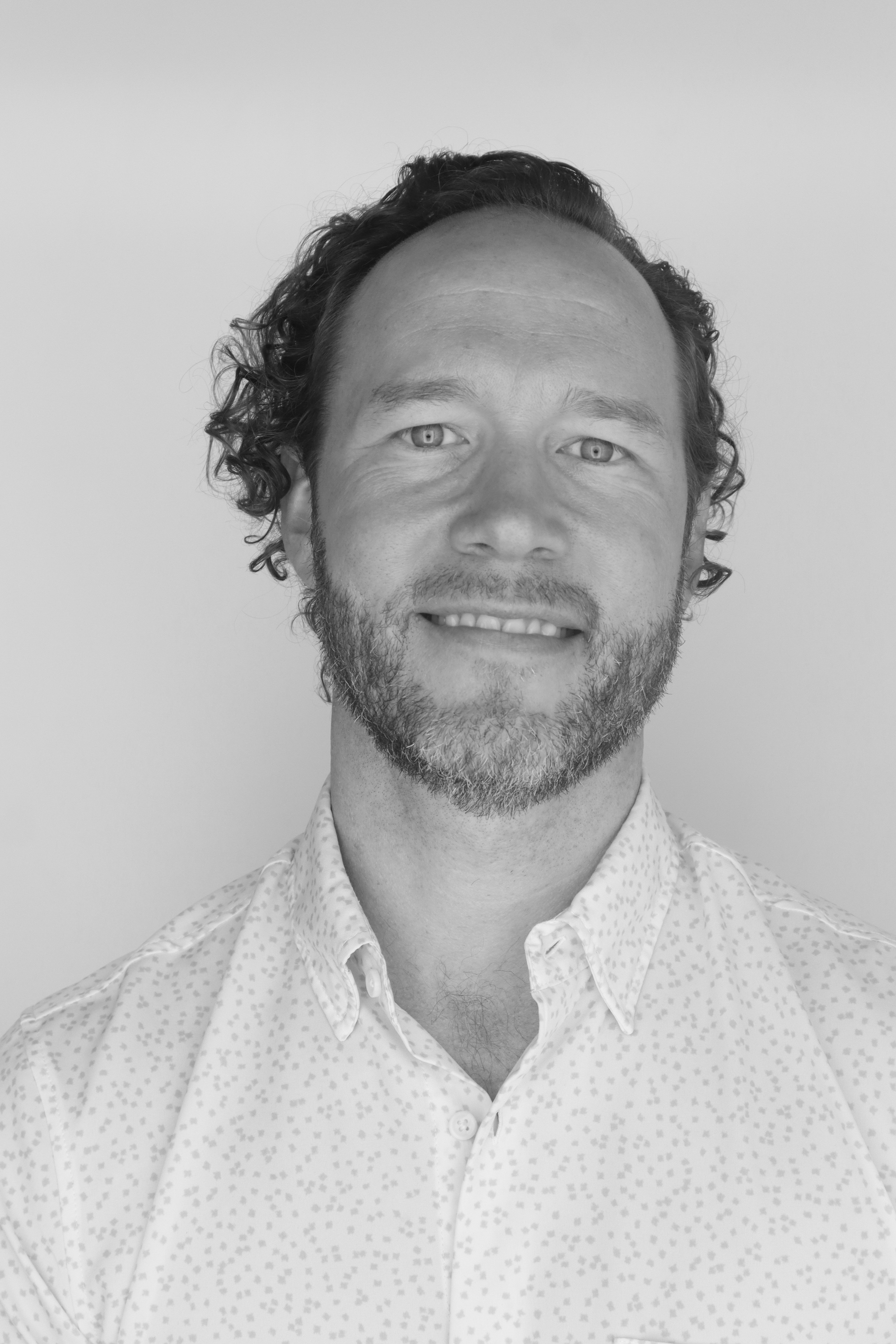