The goal of this project is to use proprioception, localized sensing, and observed forces to develop robust, autonomous fruit picking methods. Fresh market tree fruit growers still rely on a large seasonal labor force for harvesting operations. Despite extensive research over the past thirty years, robotic harvesters are not yet commercially available. Prior work has considered manipulation a robot position control problem, disregarding the need for sensor input after physical contact with the fruit. However, when picking fruit such as apples and pears, professional pickers use active perception, incorporating both visual and tactile input about fruit orientation, stem location, and the fruit's immediate surroundings. We propose to embrace this physical contact by incorporating a rich set of in-hand sensors in an extended manipulation feedback loop with the goal of providing fine control over how the fruit is separated from the tree. To overcome the constraints of data collection in the field, we will develop a learning framework for compartmentalizing the tasks and design an instrumented proxy to serve as a training environment.While our primary focus in this project is fresh market apple and pear harvesting, we believe that this framework will be useful for numerous other agricultural applications that involve physical manipulation. For example, harvesting methods used for greenhouse sweet peppers and tomatoes are highly dependent on knowledge of peduncle orientation. However, automating production has been difficult due to similar challenges with occlusions and determining crop orientation. Another potential area of application for this learning framework is plant phenotyping, using soft tactile sensors, in addition to other sensor types, to measure a plant's physical properties.
Off
Oregon State University
-
USDA
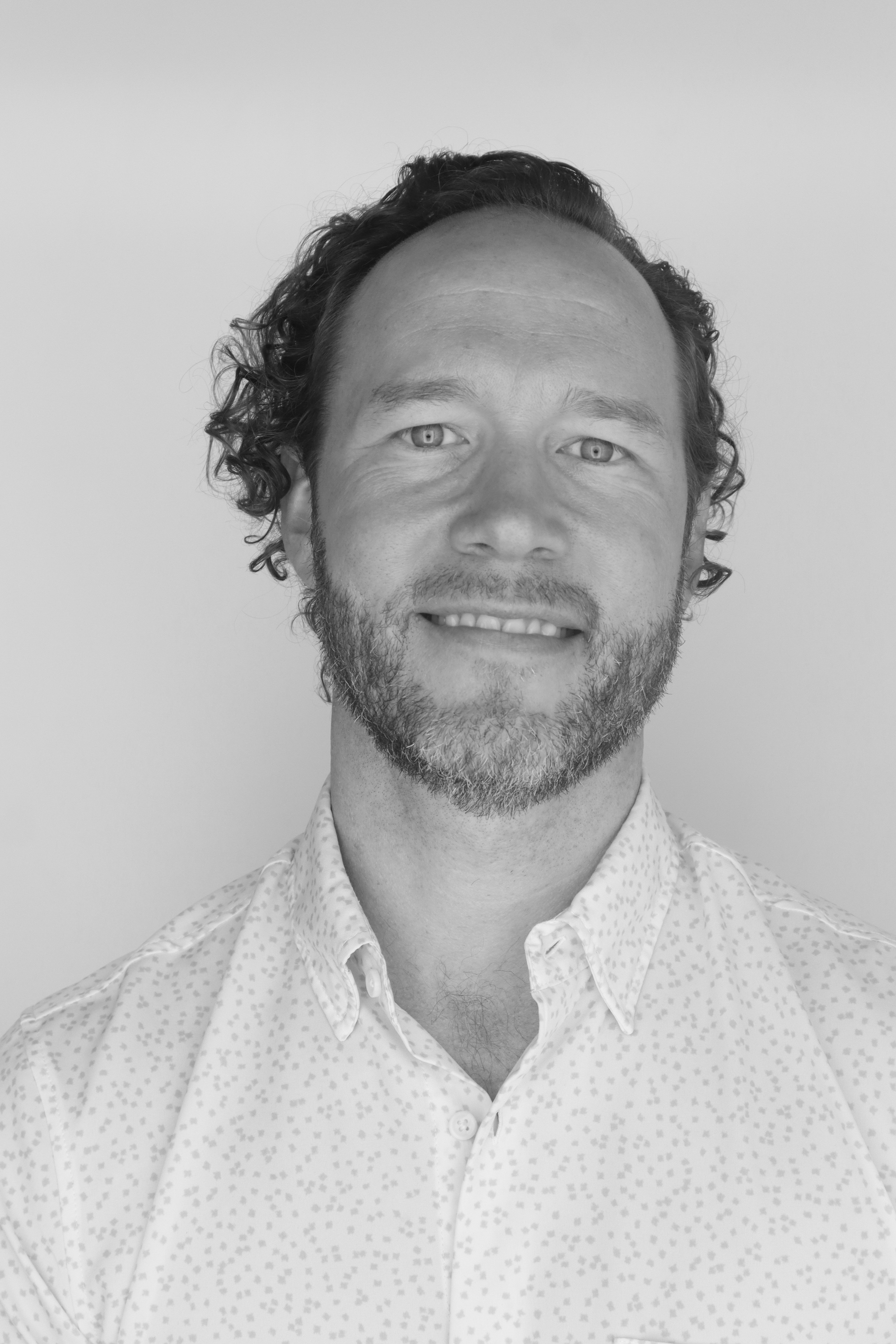