Plant diseases pose an increasing threat to the nation's food supply and biosecurity in a changing environment. There is a growing risk of plant pathogens spreading through shipments of stock plants between production facilities, and from production facilities to growers or to retailers and consumers. Recent failures to prevent plant disease emergence and spread in the US has resulted in major economic losses (20-40% of total yield) for growers. One central challenge to preventing accidental pathogen dissemination and disease outbreaks is that many plant diseases are difficult to detect at an early stage and infected (possibly asymptomatic) plants can spread pathogens undetected when being shipped from one location to another. Once an emerging disease takes foothold in a new environment, eradicating such disease is extremely challenging.As our model system, we focus on disease detection and control in transplant facilities, which produce seedlings that will later be planted in production fields. These facilities are an amplifier of diseases in the agriculture production chain because of the use of greenhouses with limited environmental control and high plant density (average 800 plants per square meter, growing on plastic trays), that facilitate disease spread. A typical transplant facility grows over 500,000 plants at a time; therefore, manual scouting of the facility is time consuming and ineffective. Poor training in plant pathology and tight schedules of employee limit sensitivity of disease detection and spatial-temporal resolution. In summary, the transplant industry is a major source of disease outbreaks and can thus be a key point for disease control in the agriculture supply chain. To reduce the contribution of transplant facilities to disease outbreaks, we plan to develop a novel disease detection and control CPS to provide closed-loop disease control at a very early stage.This project includes three main objectives. First, an open-source robotic gantry system will be developed to automate the process of greenhouse scouting, plant sampling and plant removal to prevent disease spread. Second, a microfluidic device will be implemented to automate the process of library preparation from bacterial DNA extraction, amplification to metagenomic sequencing. Third, controlled experiments will be performed to determine the best methods for disease detection via nanopore, meta-genomic sequencing, machine learning, and computer vision. Different control strategies will be tested to determine the best approach that integrates data from metagenomic sequencing and the analysis of images of infected plants.The immediate goal of our project is to reduce the spread of plant diseases in the agriculture production chain by focusing on reducing disease instances in the transplant facilities and greenhouses. Our approach is to reduce the loss of plant products by optimization of disease detection and control strategies through robotics and automated genetic sequencing. In the long run, our project will contribute to the improvement of automation and reduction of the labor cost in agriculture. Our system, if successful, will reduce the loss of plants due to diseases for greenhouse operations and in plant production systems that utilizes transplant facilities.
Off
Virginia Polytechnic Institute and State University
-
USDA
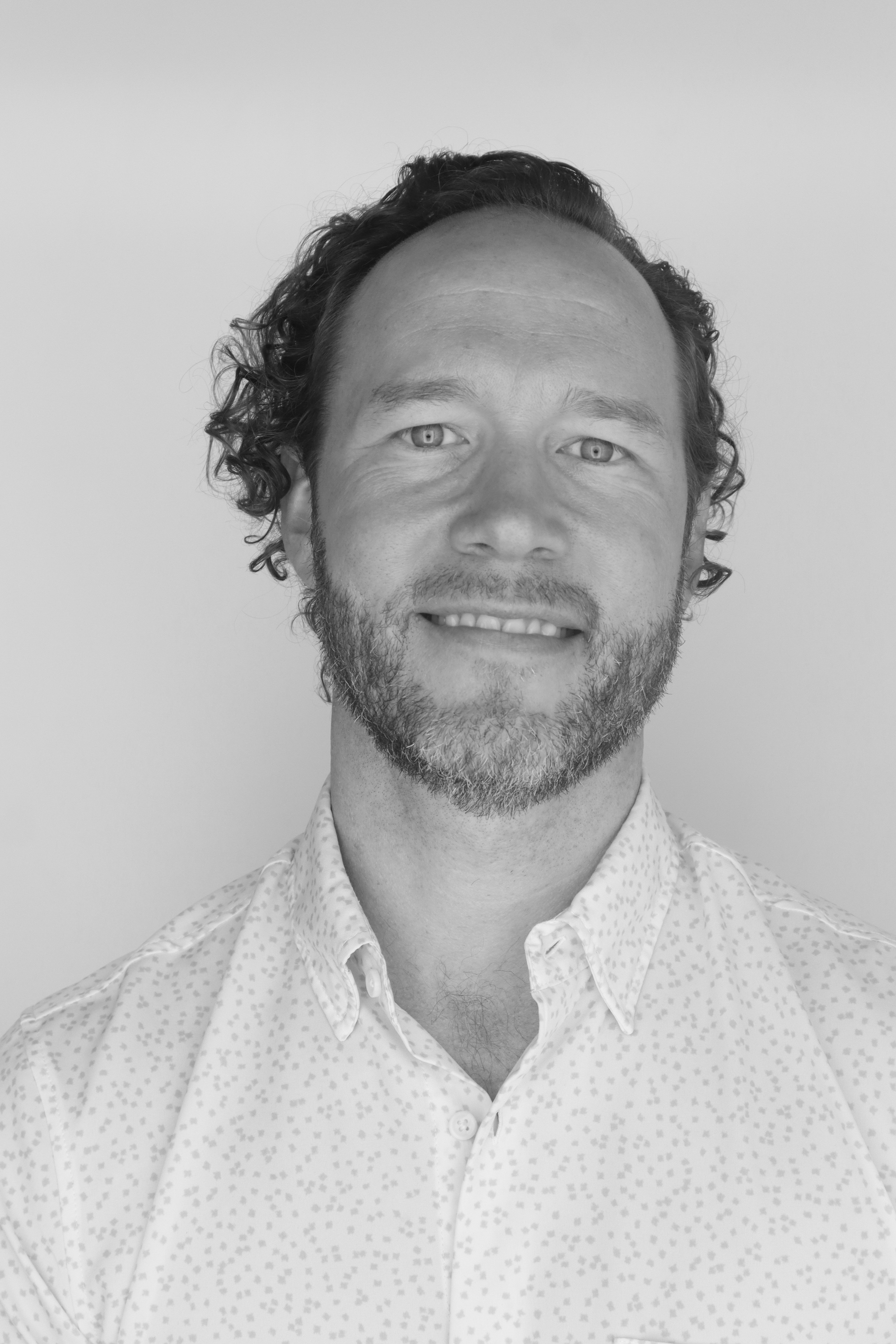