The terms denote educational areas that are part of the CPS technology.
The focus of this project is the efficient implementation of multiple control and non-control automotive applications in a distributed embedded system (DES) with a goal of developing safe, dependable, and secure Automotive CPS. DES are highly attractive due to the fact that they radically enhance the capabilities of the underlying system by linking a range of devices and sensors and allowing information to be processed in unprecedented ways. Deploying control and non-control applications on a modern DES, which uses advanced processor and communication technology, introduces a host of challenges in their analysis and synthesis, and leads to a large semantic gap between models and their implementation. This gap will be filled via the development of a novel CPS architecture by stitching together common fundamental principles of multimodality from real-time systems and related notions of switching in control theory and integrating them into a co-design of real-time platforms and adaptive controllers. This architecture will be validated at the Toyota Technical Center in the context of engine control and diagnostics.
The results of this project will provide the science and technology for a foundation in any and all infrastructure systems ranging from finance and energy to telecommunication and transportation where distributed embedded systems are present. In addition to training the graduate and undergraduate students, and mentoring a post-doctoral associate who will gain multi-domain expertise in advanced control, real-time computation and communication, and performance analysis, an inter-school graduate and an integrated summer course will be developed on control in embedded systems and combined with on-going outreach programs at MIT and UPenn for minority and women undergraduate students and K-12 students.
Off
Massachusetts Institute of Technology
-
National Science Foundation
Annaswamy, Anuradha
Project
CPS: Medium: Collaborative Research: Monitoring Human Performance with Wearable Accelerometers
The objective of this research is to develop a cyber-physical system composed of accelerometers and novel machine learning algorithms to analyze data in the context of a set of driving health care applications. The approach is to develop novel machine learning algorithms for temporal segmentation, classification, and detection of subtle elements of human motion. These techniques will allow quantification of human motion and improved full-time monitoring and assessment of medical conditions using a lightweight wearable system. The scientific contribution of this research is in advancing machine learning and human sensing in support of improved medical diagnoses and treatment monitoring by (i) modeling human activity and symptoms through sensor data analysis, (ii) integrating and fusing information from several accelerometers to monitor in real-time, (iii) validating the efficacy of the automated detection through assessments applying the state of the art in diagnostic evaluation, (iv) developing novel machine learning methods for temporal segmentation, classification, and discovery of multiple temporal patterns that discriminate between temporal signals, and (v) providing quality measures to characterize subtle human motion. These algorithms will advance machine learning in the area of unsupervised and semisupervised learning. The driving applications for this research are job coaching for people with cognitive disabilities, tele-rehabilitation for knee osteo-arthritis, assessing variability in balance and gait as an indicator of health of older adults, and measures for assessing Parkinson's patients. This research is highly interdisciplinary and will train graduate students for careers in developing technological innovations in health and monitoring systems.
Off
University of Pittsburgh
-
National Science Foundation
Redfern, Mark

Project
CPS: Medium: Collaborative Research: Monitoring Human Performance with Wearable Accelerometers
The objective of this research is to develop a cyber-physical system composed of accelerometers and novel machine learning algorithms to analyze data in the context of a set of driving health care applications. The approach is to develop novel machine learning algorithms for temporal segmentation, classification, and detection of subtle elements of human motion. These techniques will allow quantification of human motion and improved full-time monitoring and assessment of medical conditions using a lightweight wearable system. The scientific contribution of this research is in advancing machine learning and human sensing in support of improved medical diagnoses and treatment monitoring by (i) modeling human activity and symptoms through sensor data analysis, (ii) integrating and fusing information from several accelerometers to monitor in real-time, (iii) validating the efficacy of the automated detection through assessments applying the state of the art in diagnostic evaluation, (iv) developing novel machine learning methods for temporal segmentation, classification, and discovery of multiple temporal patterns that discriminate between temporal signals, and (v) providing quality measures to characterize subtle human motion. These algorithms will advance machine learning in the area of unsupervised and semisupervised learning. The driving applications for this research are job coaching for people with cognitive disabilities, tele-rehabilitation for knee osteo-arthritis, assessing variability in balance and gait as an indicator of health of older adults, and measures for assessing Parkinson's patients. This research is highly interdisciplinary and will train graduate students for careers in developing technological innovations in health and monitoring systems.
Off
Carnegie Mellon University
-
National Science Foundation
Hodgins, Jessica

The objective of the proposed research program is to develop, for the first time, the theory and methods needed for the design of networked control systems for chemical processes and demonstrate their application and effectiveness in the context of process systems of industrial importance.
The proposed approach to achieving this objective involves the development of a novel mathematical framework based on nonlinear asynchronous systems to model the sensor and actuator network behavior accounting explicitly for the effect of asynchronous and delayed measurements, network communication and actuation. Within the proposed asynchronous systems framework, novel control methods will be developed for the design of nonlinear networked control systems that improve closed-loop stability, performance and robustness. The controller design methods will be based on nonlinear and predictive control theory and will have provable closed-loop properties.
The development and implementation of networked control methods which take advantage of sensor and actuator networks is expected to significantly improve the operation and performance of chemical processes, increase process safety and reliability, and minimize the negative economic impact of process failures, thereby impacting directly the US economy. The integration of the research results into advanced-level classes in process control and the writing of a new book on ``Networked Process Control'' will benefit students and researchers in the field. The development of software, short courses and workshops and the on-going interaction of the PIs with an industrial consortium will be the means for transferring the results of this research into the industrial sector. Furthermore, the involvement of a diverse group of undergraduate and graduate students in the research will be pursued.
Off
University of California-Los Angeles
-
National Science Foundation
Christofides, Pangiotis

The objective of this research is to meet the urgent global need for improved safety and reduced maintenance costs of important infrastructures by developing a unified signal processing framework coupling spatiotemporal sensing data with physics-based and data-driven models. The approach is structured along the following thrusts: investigating the feasibility of statistical modeling of dynamic structures to address the spatiotemporal correlation of sensing data; developing efficient distributed damage detection and localization algorithms; investigating network enhancement through strategic sensor placement; addressing optimal sensor collaboration for recursive localized structural state estimation and prediction.
Intellectual merit: This innovative unified framework approach has the potential of being more reliable and efficient with better scalability compared to the current state-of-the-art in structural health monitoring. The proposed research is also practical as it allows analysis of real-world data that accounts for structural properties, environmental noise, and loss of integrity over sensors. Probabilistic representation of significant damages allows more informative risk assessment.
Broader impacts: The outcome of this project will provide an important step toward safety and reliability albeit increasing complexity in dynamic systems. New models and algorithms developed in this project are generic and can contribute in many other areas and applications that involve distributed recursive state estimation, distributed change detection and data fusion. This project will serve as an excellent educational platform to educate and train the next generation CPS researchers and engineers. Under-represented groups such as female students and Native American students will be supported in this project, at both the graduate and undergraduate levels.
Off
Oklahoma State University
-
National Science Foundation
Cheng, Qi





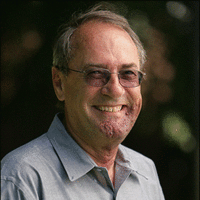